In a rapidly changing AI tech landscape recognizing an outstanding and scalable AI product as a Venture Capitalist, requires a comprehensive assessment of various factors. Here are four key considerations for identifying exceptional AI products in the early stages of startup development.
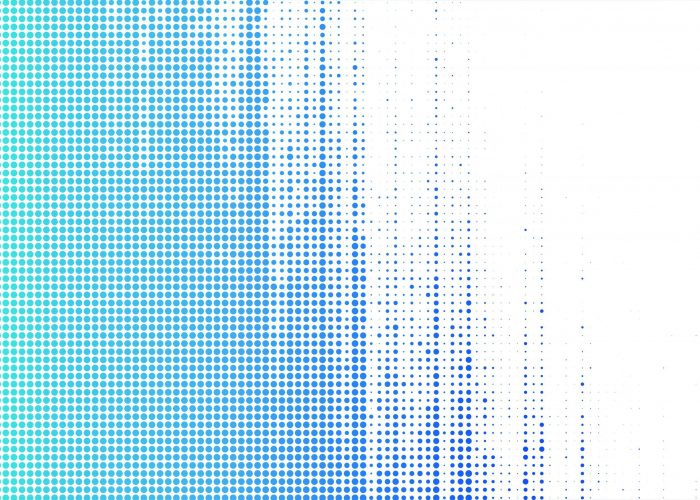
Competetive tech advantage
To recognize an AI competitive advantage, VCs and Angels must be able to recognize the tech advantage even at an early stage. What makes the AI model of a certain startup unique? What is the technical USP? How difficult is this technology for others to replicate?
Look for startups that have developed proprietary algorithms, models or unique approaches to solving use case specific AI problems. Those can provide a significant advantage by offering superior performance, efficiency, or accuracy compared to existing solutions. Evaluate the novelty and potential impact of the algorithms, and consider whether they have the potential to disrupt or reshape the industry they operate in.
Examine whether the startup has access to unique or exclusive data sources that are difficult to replicate. Unique data can provide valuable insights and enable the AI model to deliver more accurate and robust results. Additionally, partnerships with data providers or industry experts can give the startup a competitive advantage by providing them with privileged access to relevant datasets or domain expertise.
Divers data and data sources
the importance of diverse data and diverse data sources cannot be overstated. Diversification of data ensures that AI models are trained on a wide range of examples, representing different demographics, contexts, and scenarios. This inclusivity helps to minimize biases and enhance the fairness and accuracy of AI systems
Divers data source portfolios mitigate the risks associated with dependencies on specific data sources. Relying heavily on a single data source can introduce vulnerabilities and biases into the AI model, as it may not capture the full range of variability and complexities present in the real world. By diversifying data sources, startups can reduce the impact of potential biases or limitations inherent in any one dataset. Additionally, in situations where a single data source becomes unavailable or unreliable, having alternative sources allows the AI product to continue functioning and providing value.
Owned Tech Stack
Owning the AI tech stack is crucial for reducing dependencies on other companies and maintaining control over the technology itself. By owning the entire tech stack, startups have the freedom to make strategic decisions, customize the AI product according to their specific needs, and maintain control over its development and evolution. Depending heavily on third-party technologies or APIs can introduce risks such as unexpected changes in pricing, licensing restrictions, or limited access to critical updates or improvements. Having ownership over the tech stack allows startups to iterate quickly, experiment with new features, optimize performance, and respond promptly to customer feedback. It also provides the flexibility to integrate new technologies or adapt to emerging trends without being constrained by the limitations or business decisions of external providers.
User Input used for Machine Learning
The incorporation of user input, such as prompts, engagement, and feedback, is of paramount importance for improving AI models through machine learning. User input serves as valuable training data that helps refine and enhance the model’s performance over time.
Strong AI start-up teams build models that actively involving users in the learning process. By doing this, they can gather insights, preferences, and real-world context that might not be adequately captured by existing datasets alone. Incorporating user input as a feedback loop can lead to a continuously refinement of AI models, increase accuracy, and deliver better outcomes aligned with user needs and expectations. Ultimately, user input-driven machine learning fosters a dynamic relationship between the AI product and its users, resulting in a more user-centric and effective solution.